Why Double Checking Generative AI Outputs is a Silent Productivity Killer
Is your AI tool a helpful assistant or a demanding supervisor? Discover the hidden cost of double-checking AI-generated content and its impact on your productivity.
ARTIFICIAL INTELLIGENCE
7/28/20244 min read
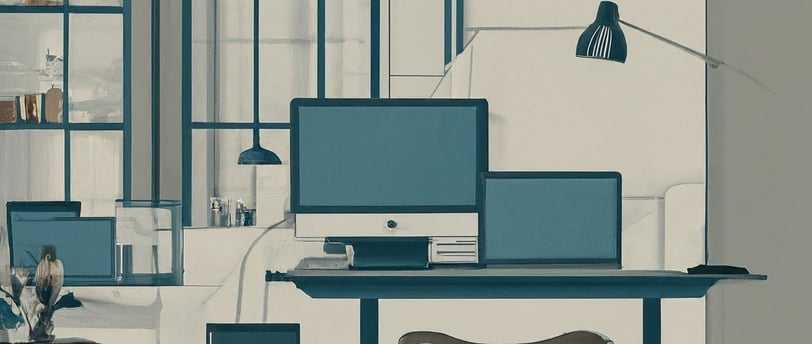
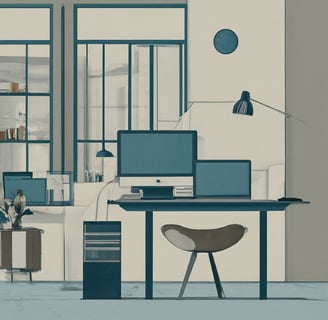
We not only use AI tools like chatbots but also help build new ones for specific use cases. For every response we get from a language model, we need to double check it. This burden is actually common to many workplaces that use these tools on a daily basis and is slowly becoming a driving force behind the AI burnout.
One of the projects we worked on was how to enhance an applicant’s CV according to a job description to improve diversity across the applicant pools. It would make it easier for the applicants to get feedback and feel more confident in applying to jobs they are interested in as opposed to what their CV can get. For example, the system can tell them to reorganise sections or suggest things to add.
Did it work? Like most large language model based projects, there are cases where it works well and there are cases where it doesn’t. How do we know this? Because we had to check the outputs it gives, try to verify the responses and tweak the process. This is the hidden cost that drains the productivity out of the team, creating a cycle of generate and check.
The Double Checking Dilemma
People are quite aware that large language models can hallucinate. But more often, it is the tone of the reply such as “Yes, sure, absolutely, I can help you improve your CV!” which sounds like an 8-year old feigning to give careers advice or the lack of material value such as “The CV looks really nice! I suggest you swap the Education section with Experience.” While your customer is hoping for a valuable answer, they often have to manually parse the response to see if there is anything useful.
The dilemma is clear: AI is meant to save time, but the double checking process can be very time consuming. As soon as we start spending more time checking the result of a generative AI output rather than actually doing the task ourselves, we have a major problem. For example, instead of writing this post ourselves, we could have generated it a minute giving a language model the section headers but probably spent at least 30 minutes combing through the generic output. On the other hand, writing the post in 45 minutes guarantees a valuable, correct and unique output.
Checking the output of generative AI is akin to browsing the internet in the age of digital advertising. We have to learn how to deal with the subpar, incorrect, childish and verbose outputs bombarded at us from almost every product and service we use today.
We recommend reducing the scope of generative AI. The more ambitious and the generic the application the more room there is for things to spiral into the land of uselessness. For example, categorising documents across a given set of categories automatically might work well and limit the scope nicely. However, asking a language model to generate documents for each of those categories is a burden for a poor person to check what it has generated.
The Psychological Impact
When we trust a source of information such as a reputable news outlet, we consume that information and move on with our day. We don’t fact check, or question the reliability and that’s why most reputable news outlets charge for their content. Whereas AI generated content comes straight at us with a set of half-baked filters for example when a chatbot says it is a language model and cannot help.
Is this output correct? Should I trust this result? I know AI generated this content but should I present it in the next meeting? While there are positive outcomes of generative AI across a wide range of tasks, the mental burden of vigilance can lead to decision fatigue. The more we use AI tools, the more times we have to parse, check and refine the output.
Sometimes instead of acknowledging the limitations of these tools, people blame themselves for not being able to prompt correctly or go down a rabbit hole of trying to get the AI to do the task rather than doing it themselves. This phenomenon is more common in generating text. People start with a prompt, then refine the tone, then ask to add something or remove a phrase, then generate more examples until the interaction loses its original intent.
In these cases, we suggest taking a break from generative AI and try different tools. It is unfortunate that due to the amount of investment into AI, it is marketed as something people cannot or will not be able to live without whereas with many things, generative AI is another tool for a downstream task.
Solutions and Strategies
Generative AI is often a tool and not the solution. There is always an underlying task such as providing better customer support or improving job applications. Going back to the problem statement can help seeing different avenues and areas of innovation. There are many other applications and methods of machine learning that could be useful to the problem. At Semafind, we try to provide a bigger picture and recommend solutions that may or may not include language models, image generators and so on.
Allocate a budget and be aware of the resources, including time spent. While the immediate marketing value can be tempting, we observe that integrating or using generative AI for the sake of doing it usually leads to more headache and stress let alone having more frustrated customers who have to speak to chatbots. The damage of a chatbot can be significant to the reputation of the brand and to the trust of its customers.
We predict that over time, more people and businesses will get accustomed to the limitations of generative AI and adapt their behaviour accordingly. It may well be with increasing AI usage, businesses who can offer genuine human interactions and support could become more attractive.
Legal
© 2024 Semafind Limited. All rights reserved.
Company
Semafind Ltd.
36 Bruntsfield Place, Edinburgh, United Kingdom, EH10 4HJ
SC745698 - VAT GB435273890